Machine learning for predictive maintenance
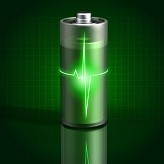
Predictive (or positive) maintenance is a major challenge for the railway sector. The "Artificial Intelligence and Positive Maintenance" research initiative supported by Europorte (Getlink Group) is innovative in its approach through the analysis of a large amount of data with an approach to making industrial activity more reliable. For example, by prolonging the life of batteries, we can considerably reduce their risk of failure and thus make their use more reliable. Although charging protocols are one of the main factors influencing the life of batteries, they remain little studied from an industrial point of view, despite their positive economic and ecological impacts.
This topic is at the heart of the work of Ahmed Shokry, a research engineer at the École Polytechnique as part of the research initiative. With the help of Éric Moulines, programme holder and professor at the École Polytechnique's Centre for Applied Mathematics (CMAP*), Ahmed is developing algorithms based on machine learning to control and optimise the charging of the most commonly used lithium batteries.
These algorithms, organised in neural networks (so called by analogy with the functioning of the brain), make it possible to achieve an optimal balance between the speed of charging and maintaining the health of the battery in the long term. To achieve this, they integrate multiple sources of information to develop precise and computationally light control laws. These laws can be installed on the battery management system to control the charge based on real-time measurements of the battery's condition, such as capacity, voltage and temperature.
The use of deep artificial neural networks to address battery charge control is a novel approach. Indeed, these problems are usually solved using mathematical optimisation methods which, in addition to human effort, require significant online computing resources that are not available on most industrial battery management systems.
Ahmed Shokry's work therefore introduces a new and practical approach to battery charge control, expanding the range of solutions available and enabling the commercialisation of this technology. His work within the research initiative is also leading him to develop machine learning techniques to detect early signs of battery degradation, as well as all locomotive safety components, which will allow predictive maintenance.
*CMAP: a joint research unit CNRS, École Polytechnique - Institut Polytechnique de Paris
>> About the research initiative:
The "AI and Positive Maintenance" research initiative, led by Éric Moulines, seeks to anticipate hardware or network failures. To meet this challenge, it is necessary to make sense of the weak signals that can be observed in a large amount of data. Supported since its creation in 2020 by Europorte, the research initiative is studying this problem from the perspective of AI methods capable of massively processing data. These AIs will use new machine learning and deep learning methods to reliably detect weak signals.